

Frequent itemset mining was first proposed in Agrawal, Imieliński, and Swami (1993) for market basket analysis in the form of association rule mining. The underlying rational is that the higher the support, the more interesting the itemset. The majority of the existing interestingness measures are based on support, which measures the number of transactions that contain the entire itemset. As itemset mining has been researched for about two decades, a number of interestingness measures have been proposed and studied (Geng & Hamilton, 2006). Indeed, interestingness measure plays an important role in itemset mining, as it determines the knowledge to be extracted and impacts the time and space costs of the mining process. The goal of itemset mining is to retrieve all interesting itemsets with respect to some interestingness measure, which is used to select and rank itemsets according to their potential value to the users. people buying onions and potatoes are more likely to buy burger this can be utilized for marketing purposes. Interesting itemsets can be further used to induce patterns like association rules, e.g.
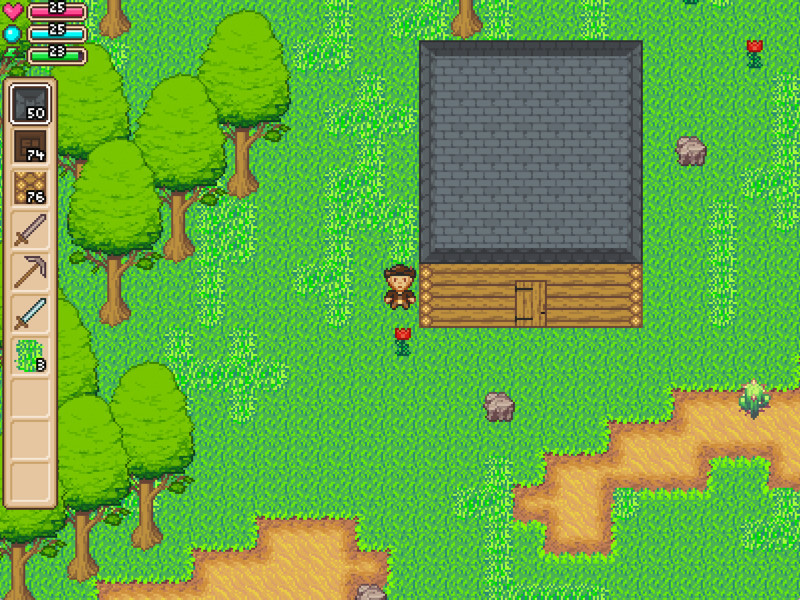
Interesting itemset mining, as a fundamental research problem in data mining, is to extract knowledge, which is formed as sets of items, from a transaction database that consists of transactions of items and can be represented by a binary matrix. Our experimental results show that our algorithms can effectively analyze real datasets and retrieve meaningful results.
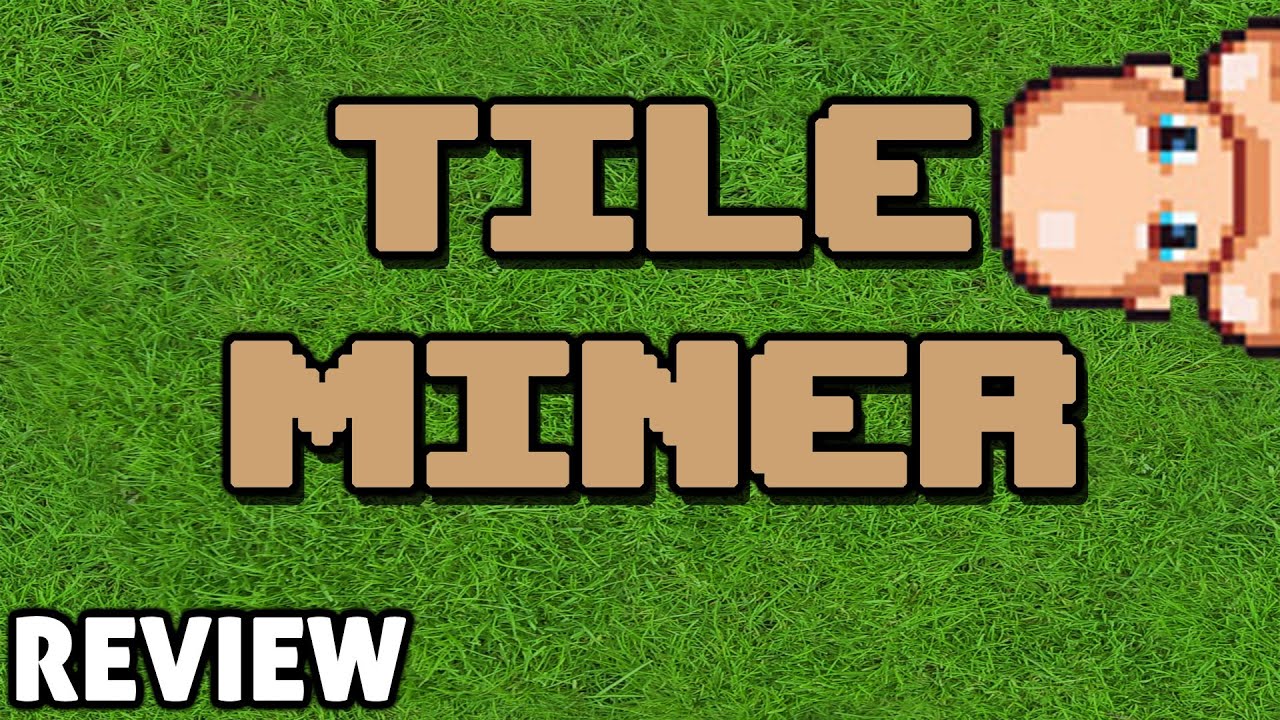
To tackle the challenge, we utilize the branch-and-bound search strategy to analyze the characteristics of candidate itemsets at each searching branch and estimating their bounds. While using some monotonic property to prune search space is a common strategy for interesting itemset mining, no monotonic property is available for this problem. Due to the exponential search space of candidate itemsets, both problems are NP-hard. Given a user-defined fault tolerance value, we are interested in finding the maximum/top-k fault-tolerant tiles. extracting fault-tolerant association rules, analyzing noisy datasets). That is based on two observations: (1) the length of an itemset can be as important as its frequency (2) knowledge discovery from real-world datasets calls for fault-tolerant data mining (e.g. This paper proposes a new interestingness measure, the fault-tolerant tile. It is intended to identify interesting relations between variables in a database using some measures of interestingness and has a number of applications, including market basket analysis, web usage mining, intrusion detection, and many others. Interesting itemset mining is a fundamental research problem in knowledge management and machine learning.
